Autonomous self-driving cars keep inching ever closer to reality. More companies are getting into the self-driving game, but one big problem remains: Self-driving cars have a really difficult time identifying people on bikes. And they are even worse at predicting where those cyclists are headed.
Autonomous cars & their issues identifying cyclists
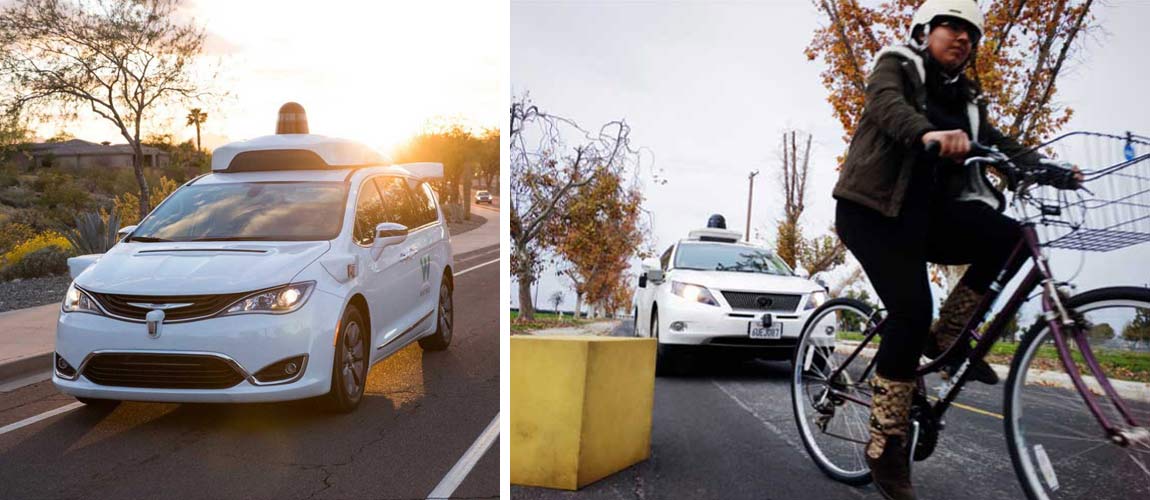
A couple of new reports out since the start of 2018 have summarized the huge progress that self-driving car technologies have made in the last year or two. On one side industry group IEEE has credited progress overall, but stagnation regarding cyclists. And then there is Waymo – the evolution of Google’s self-driving car project – that released a safety report showing the strides they’ve made, specifically calling out cyclist detection.
These autonomous vehicles are becoming smarter and much more accurate at identifying, tracking, and even predicting the movement of objects in their field of view. The problem remains, though, that progress doesn’t appear to be being made with regards to cyclists.
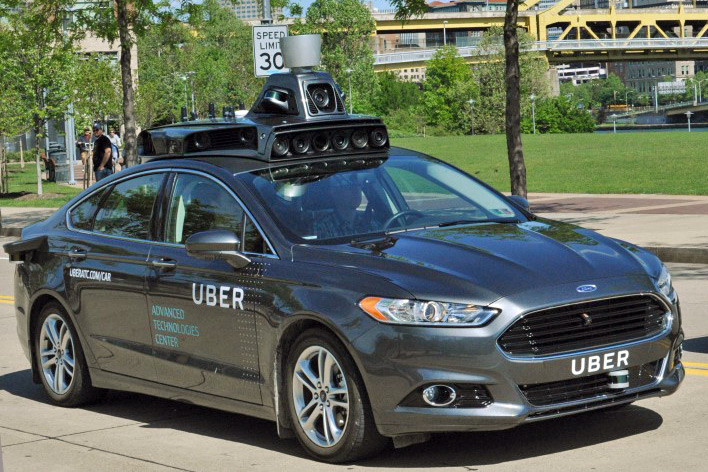
We reported on the same issue a little over a year ago when Uber admitted their own self-driving cars were having trouble identifying bike lanes. That made it difficult, both to keep the cars out of the lanes and to recognize that they should be carefully looking there for cyclists.
Cyclist detecting technologies

And a lot of that comes down to the most common technology that self-driving cars utilize – namely analyzing a constant inflow of two dimensional images to identify objects and their direction of travel. Many vehicles combine that optical sensing with additional tech like lidar & radar which help identify locations of objects. But those sensing systems are less effective at determining their direction of travel, limiting their effectiveness on their own.
Essentially the autonomous car sees the world as a succession of images, and software automatically models boxes around things like cars (green & purple), pedestrians (yellow), and cyclists (red) over the vehicle’s 3D basemap data.
Then it anticipates what happens next, so it can decide how to proceed driving.
Cyclists are a difficult thing to identify and track. We are less massive than other vehicles on the road. Plus, we are able to both move speedily with traffic and react quickly, changing direction faster than almost anything else in the roadway.
But maybe the biggest obstacle for the automated machine analysis made by self-driving cars is that cyclists are so individual. Whether we are roadies in skin tight lycra, mountain bikers in baggies, or a commuter in regular street clothes, we all look very different, even though we move in similar ways. While autonomous cars have effectively learned how to identify mostly standard looking cars by studying huge numbers of images, those images haven’t had a lot of cyclists in them, so there is much more learning to be had.
How can self-driving cars and cyclists co-exist?

Some cycling & car industry minds have been developing technologies, using reflective paint, specially identifiable helmets, a smartphone app, and even bikes equipped with technology that communicates directly with the self-driving cars. That seems like a band-aid over the problem to us (and many others). It certainly could help those equipped with the special tech, but would ultimately leave anyone without it more vulnerable.
For now it seems like the best solution is more machine learning. The programmers behind the development of these self-driving cars need to get more images of cyclists into the robot brains of their cars, so they can make the right decisions.
The same problems exist with regular drivers who don’t always react properly to seeing cyclists on the road. (Not helped by some cyclists not obeying the rules of the road to start with.) Much like we need to use cycling advocacy to teach human drivers how to share the road, it seems we need to do the same with self-driving cars. And as more autonomous vehicles encounter more cyclists out on the road, they can better learn to identify us and operate safely around us.